Welcome to our quarterly newsletter. We present you with a few updates: to our IFRSX product, stress testing and also to our new ML platform YQ. Next-up, Rado brings an in-depth blog on Why MLOps is the way forward for maintainable data products.
A few introductory words from David:
The current economic crisis is a mixed blessing for software companies. It presents challenges, but also opens new opportunities for working remotely in the Cloud and for finding skilful IT experts.
At CREDO, we are investing in the future by improving our existing tools and building new products. We will soon welcome two new colleagues that will help us realize these ambitions. We are also making our first steps across the Atlantic, in Latin America.
Let’s hope we can all see each other as much as possible in person in autumn in the data science and financial community. Otherwise a good opportunity for us to meet is virtually, in our upcoming webinar on “Effective Modelling in the Cloud for Financial Institutions, using Kubeflow” on 24 September!
IFRSX – latest improvements
Based on an interesting round of “Voice of the Customer” workshops and specific user requests, we have extended the functionalities of our existing IFRSX tool. These optional features are now accessible to all users .
The following improvements are available in the latest IFRSX release:
- The Management overlay can be applied in a relative way now, by applying a factor on the ECL amount calculated by the tool, whereas in previous versions only the new stage or a new ECL amount could be applied.

- To save space in the working environment and database of the tool, results can now be moved to a long-term archive. Once archived, they are stored in the ‘Parquet’ Big Data format and can be queried via the appropriate tools. Moving results to the long-term archive can be done either via the GUI or using a set of given rules.
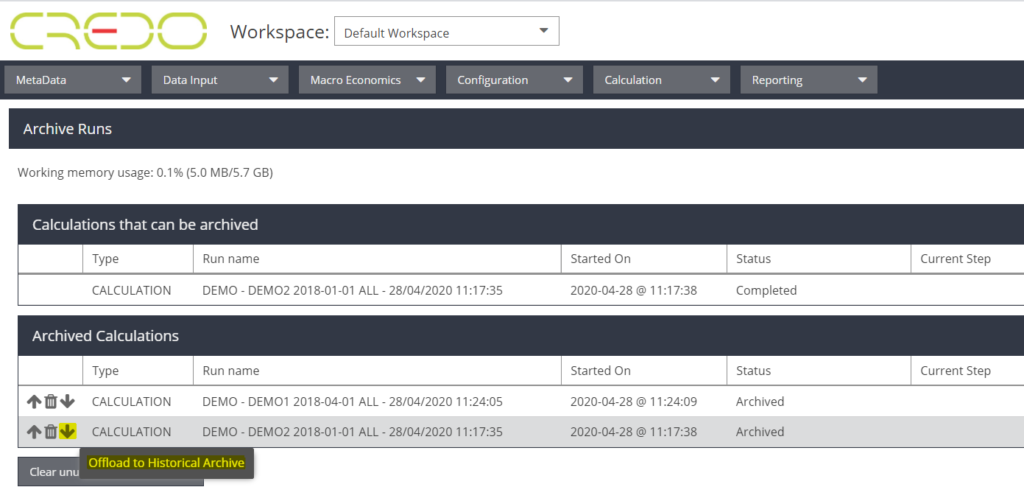
- A large part of 2019 was devoted to improving the LGD modelling capabilities in IFRSX. The latest version offers the possibility to apply unsecured recoveries in the calculation of the expected loss. This means the given model will recover potential residual unclaimed asset value, totally or partially.
- Besides these functional extensions, we also improved the user experience by making some changes in the user interface.
As of
reporting date 30/06, the impact of COVID-19 should be clearly visible in the
IFRS9 ECL and stage transfer calculations of our customers. Do not hesitate to
contact us for technical or modelling advice, if needed.
We have received some suggestions to extend the IFRS9 module with auditable Basel PD/LGD/EaD calculations and would be glad to have your opinion on this.
IFRS16 Additions
Also the IFRS16 disclosure functionality has been further improved.
- It has a dedicated screen
- The user no longer needs to re-run all the calculations in scope
Stress testing and scenario analysis – work in progress
Direct feedback from European and national regulators confirms our expectation that, as of 2021, stress testing and scenario analysis will play a bigger role in the lives of Risk Managers.
Both streamlining existing processes and replacing high level aggregate calculations by analyses based on more granular data will be key to this ‘new normal’. Together with this, we notice that the existing IFRS9 module is already used intensively for ‘what if’ analysis today – a purpose it was not originally intended for, then again, why not?
We therefore continue extending our IFRSX platform to a broader tool for integrated risk calculations, including granular, dynamic balance sheet evaluations. In the meantime, have you considered the following useful tips & tricks for facilitating your stress testing runs using recent versions of IFRSX?
- There is no need to reload and overwrite the history of risk drivers in the tool to ‘modify the past’ in your stress tests. Instead, simply load forward looking scenarios that start sufficiently in the past and make sure that the ‘validity date’ of these scenarios also lies in the past. IFRSX will apply the forward looking scenarios as of the validity date for you.
- Don’t erase and reload all risk drivers for each analysis that you are doing. Instead, add extra forward looking scenarios with risk driver names that clearly refer to the analysis they are meant for. The audit trail should capture extra uploads and changes made to these scenarios.
- Do not set up several runs in case all you want to do is analyse the impact of model parameters such as different CCF values, recovery rates, etc. Instead, do a single run using a model config with several ‘model scenarios’, each of which contains a change in model parameters.
Do not hesitate to contact us in case something is still unclear, or you need further help in efficiently setting up your stress tests and scenario analyses.
YQ: Customizing Kubeflow for cloud-based machine learning operations in the financial institution
There is a pressing need in the market to quickly build more robust data science pipelines and real-time web apps. Linking the creativity of data scientists (read: ever-evolving models) to the IT department’s secure production environment is key to this. Credo offers an end-to-end machine learning solution that does just that. We named it “YQ”.
YQ makes clever use of the emerging Kubeflow machine learning technology (Google, IBM, Cisco…) to make development, deployment and operations of data science activities more efficient and less costly for financial institutions. The three most important benefits it offers:
- Seamlessly develop, deploy, and operate any large number of data transformation scripts and quantitative models (data products)
- Have a one-stop shop for all model-related activities across all domains: Risk management, Finance, Actuarial Function, Digital Marketing, HR, etc.
- Harness the full power of the Cloud: performance, agility, ad-hoc scalability, and geographical independence
Feel free to contact us for more information or sign up for our upcoming Webinar!
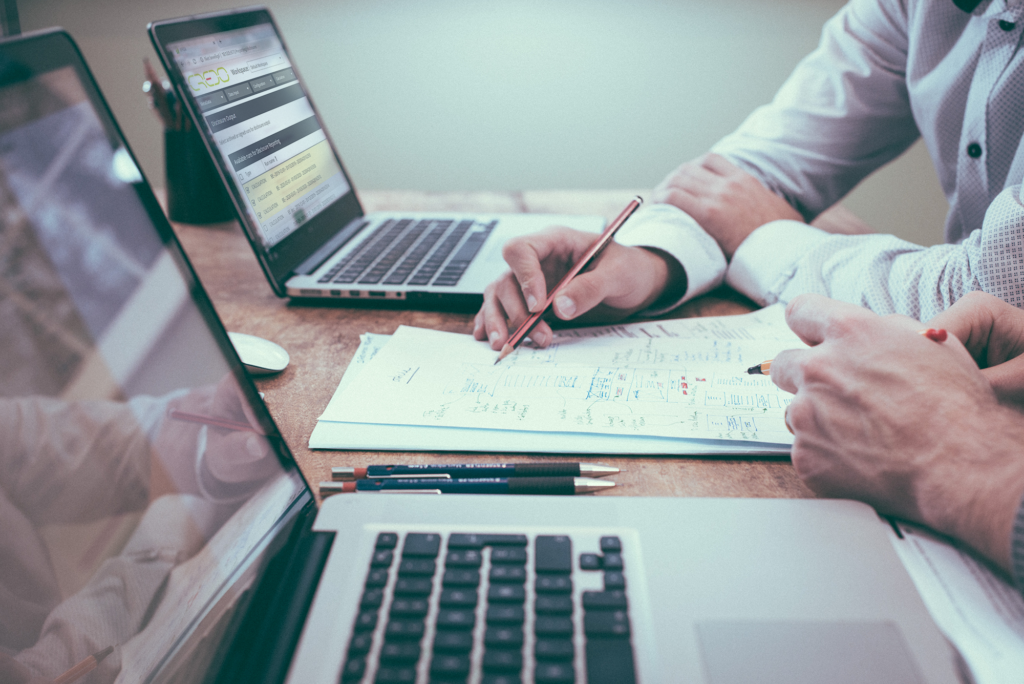
Blog by Radovan Parrak: Why MLOps is the way forward for maintainable data products
Meet ‘Machine Learning DevOps’, the newest kid on the block in a series of disruptive technologies that are set to change the world!
Also referred to as MLOps, it is a natural extension of DevOps, which has already become a mainstream efficiency and reliability booster in software development and operations. [1][2] MLOps has the same goals for the field of machine learning, a cornerstone of data science, quantitative finance and many other modelling disciplines.
Many non-tech companies in data-intensive industries (finance, telco, retail …) are still turning a blind eye to this effectiveness booster, sticking instead to manual workflows for developing and implementing their data products. I see this as a strategic error that will reduce the competitiveness of these companies in the foreseeable future. The good news is that all of this can be easily avoided using modern tooling and ways of working.
What is the real issue, and what are the risks of standing on the sidelines?
It is by now widely accepted that the ever-increasing amount of data that surrounds us contains business value, when properly mined. But from there on, paths greatly diverge: how the data is to be mined, how to exploit it in a repeatable and scalable way, how to define and measure success… One of my main observations from this debate is that many underestimate just how important it is to build ML setups in a well-thought way from the very start. It generally takes many years to come to a fully operational ML setup and thereafter the operational cost to keep it operational and up-to-date can be substantial – so some up-front thinking is not a waste of time.
Engineers at Google [3] recently identified hidden technical debt as a major issue to the domain of machine learning. In layman’s terms, the reasoning goes somewhat like this: humans are much more cunning than machines and use a lot of creativity to get fast results and ‘hack’ a solution forward. This allows entropy, or ‘technical debt’ to creep into the system. Entropy that accumulates and eventually must be accounted for with debugging, refactoring or, worse, starting over. The biggest problem with technical debt is that by the time you realize it has accumulated, it is usually too late. Non-scalable infrastructure, countless lines of legacy code, critical dependence on key persons, lack of auditability, etc., become increasingly problematic.
So what are the solutions?
Risk managers may recognize the above from their daily experiences with risk modelling for regulatory purposes. Financial regulators address this by imposing rigid model management frameworks to manage the life cycles of risk models.
Fortunately (well, at least from my point of view), similar problems occur in most other areas of predictive modelling, such as data science for marketing purposes. Precisely because the problem is so pervasive, a lot of creative thinking has been done to come to better solutions than creating telephone books full of rules. In MLOps, proven IT processes and supporting technologies go hand in hand to make all stakeholders (data engineers/scientists/analysts, IT developers, operators …) work together efficiently. By working along the principles that tools such as Kubeflow (a container-orchestration system for automating computer application deployment) impose, technical debt does not get a chance to creep in.
What is MLOps and how it emerged? Who is involved already?
“MLOps (a compound of Machine Learning and “information technology OPerationS”) is [a] new discipline/focus/practice for collaboration and communication between data scientists and information technology (IT) professionals while automating and productizing machine learning algorithms.” — Nisha Talagala (2018)
The technology industry titans are pioneering MLOps, but we are happy to see that some financial institutions are taking gradual steps to adopt this methodology too.
Where to start?
We will be coming back with a dedicated article to follow up on this topic, but a quick answer for now can be: Go cloud-native.
What are the advantages?
- Elasticity: It is hard to predict the right scale of on-premises infrastructure up front. In the Cloud, the computational capacity that you need is always available at the tips of your fingers.
- Getting up to speed: state-of-the-art MLOps tooling is readily available. For instance, a working environment based on Credo YQ can be set up in a matter of days, not months.
- Automatic updates at all levels of the stack (bare metal, OS, middlewares, data products production platform …)
- Easier and safer experimenting: under the infrastructure-as-code paradigm, it is easy to create sandboxes for experimentation
Another element to consider is how to tailor the wide-range of open-source frameworks and Cloud services (data lakes, storage services, DB services, Kubeflow, Kubernetes, GIT, Jupyter, and many more) to your specific industry and use cases.
You can learn more through following the blog series in this newsletter, but if you don’t want that long with your questions, just contact us at Credo or take part in our upcoming Webinar!
Footnotes:
[1] https://services.google.com/fh/files/misc/state-of-devops-2019.pdf
[3] https://papers.nips.cc/paper/5656-hidden-technical-debt-in-machine-learning-systems.pdf